Soumik Mandal successfully defends his dissertation.
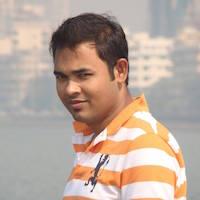
Our Ph.D. student, Soumik Mandal, has successfully defended his dissertation titled “Clarifying user’s information need in conversational information retrieval”. The committee included Chirag Shah (University of Washington, Chair), Nick Belkin (Rutgers University), Katya Ognyanova (Rutgers University), and Michel Galley (Microsoft).
Abstract
With traditional information retrieval systems users are expected to express their information need adequately and accurately to get appropriate response from the system. This set up works generally well for simple tasks, however, in complex task scenarios users face difficulties in expressing information need as accurately as needed by the system. Therefore, the case of clarifying user’s information need arises. In current search engines, support in such cases is provided in the form of query suggestion or query recommendation. However, in conversational information retrieval systems the interaction between the user and the system happens in the form of dialogue. Thus it is possible for the system to better support such cases by asking clarifying questions. However, current research in both natural language processing and information retrieval systems does not adequately explain how to form such questions and at what stage of dialog clarifying questions should be asked of the user. To address this gap, this proposed research will investigate the nature of conversation between user and expert intermediary to model the functions the expert performs to address the user’s information need. More specifically this study will explore the way intermediary can ask questions to user to clarify his information need in complex task scenarios.